If there’s one thing we know for sure – things will eventually go a bit wrong…
And when they do, it’s important to check in on what caused the problem and stop it from happening again (orrr at least to the best of our ability).
Diagnostic analytics dives into your historical data to help you uncover the root cause of your problem, allowing you to make more informed decisions about the potential solutions.
This article covers:
- What diagnostic analytics is
- How it fits into business analytics
- How it works
- Its benefits in RevOps
- 7 steps for using diagnostic analytics
- Common challenges
- Use cases for diagnostic analytics
What is diagnostic analytics?
Diagnostic analytics is a branch of analytics that examines historical data to identify the cause of an outcome. This approach to analytics aims to uncover why certain outcomes happened.
This understanding of previous data can allow businesses to identify what caused success or failure and allows them to optimize this in the future.
How diagnostic analytics fits into business analytics
Diagnostic analytics is one of the four main types of analytics used in business. The other types of business analytics are:
- Descriptive analytics: Summarizing key trends in the data set to understand what happened.
- Predictive analytics: Predicts future trends and how they might impact your org.
- Prescriptive analytics: Discusses potential actions to deal with future trends.
Diagnostic analytics typically acts as step two within this process, as once you know what occurred, you can use diagnostic analysis to uncover why it happened. Then you can look at future trends and responses to improve your org’s overall outcomes.
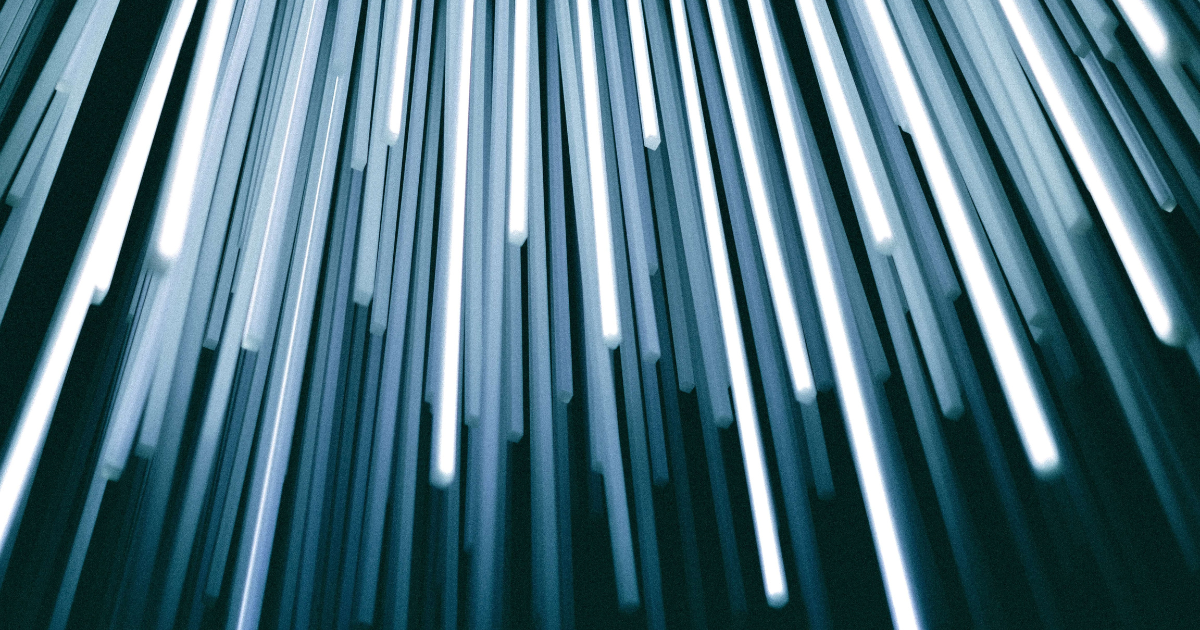
How does diagnostic analytics work?
The process of diagnostic analytics involves collecting, analyzing, and interpreting data, formulating hypotheses, and conducting tests to gain insight into the patterns within the data.
The key component of this analysis is your internal organizational data, but you may also want to utilize some external data sources to provide a well-rounded picture of the factors leading to the outcome you’re diagnosing.
There are a few different techniques for analyzing this data such as:
- Data drilling: Diving deeper into the data to gain more detailed insights.
- Data mining: Finding patterns within large databases.
- Correlation analysis: Discovering how strongly variables are linked together.
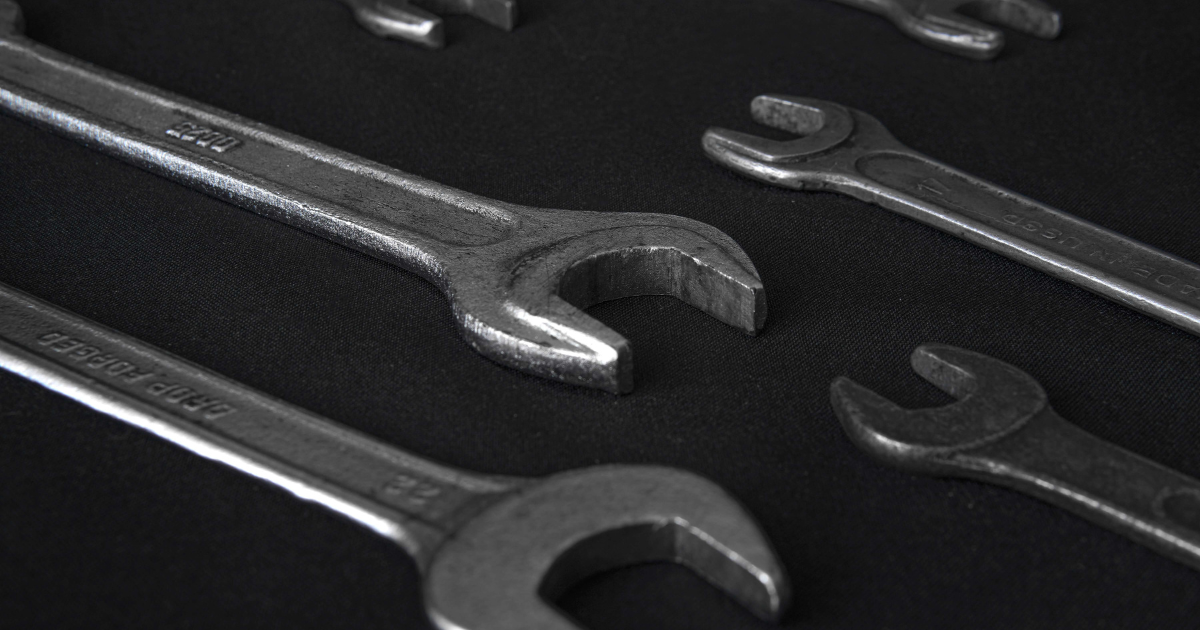
The benefits of diagnostic analytics
Diagnostic analytics offers many benefits as it helps businesses understand why certain things happened and allows insights for improvements and risk mitigation.
Enhance decision making
Utilizing this method of analytics allows for more effective data-driven decision-making as you can understand the underlying drivers for trends and outcomes in your business environment.
This information allows you to skip superficial problem-solving and dive straight to the root of the issue.
Improve process optimization
These insights also allow you to discover inefficiencies within your processes and optimize your revenue team’s workflows. For example, if prospects drop off due to a long lead time on contract approval, you may look to implement or improve your deal desk function.
Boost customer satisfaction
Diagnostic analysis can also help your teams understand your customers better as it can show trends in customer behavior and identify characteristics of your ideal buyers. Leading to improved communication, customer experience, and customer loyalty.
Reduce risk and save cost
By identifying risk early, your business can take action to minimize its impact and save from losses and additional costs that may occur without action.
Increase revenue generation
Diagnostic analysis can also highlight opportunities for growth and increased revenue generation, such as: new industry trends, new audiences, new products/services, increased efficiency, and improved customer retention.
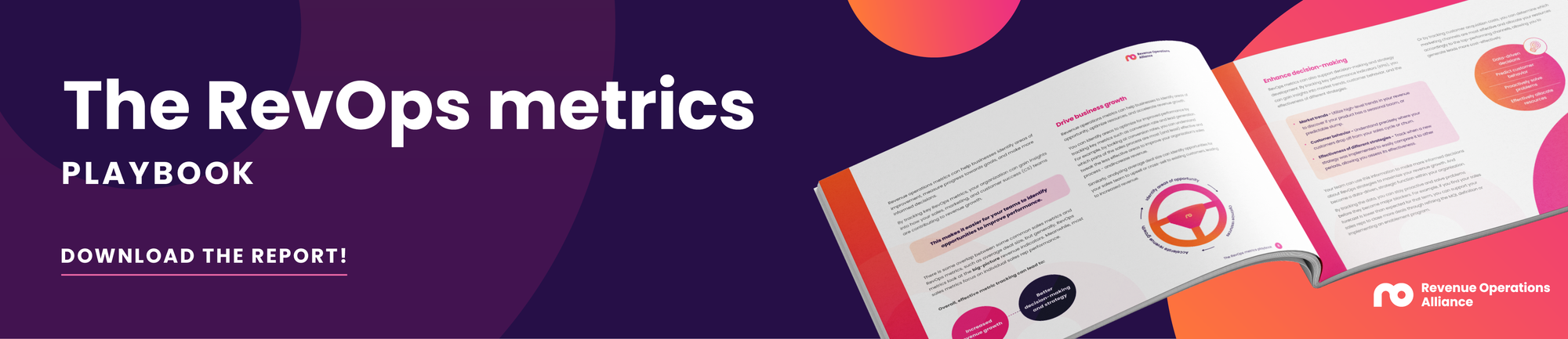
7 steps for using diagnostic analytics in your revenue strategy
The diagnostic analytics process can be broken down into the following steps:
Identify problem
The first step in diagnostic analytics is identifying the problem to be solved. Defining the problem is key, as it’ll determine the data you’ll need to collect and analyze. Having a clear idea of the problem will allow you to focus your investigation and only use relevant data, saving you time in your investigation.
Some example problems to explore might be: a decline in sales, reduced web traffic, or increased customer churn.
Data collection
Next, it’s time to collect all relevant data for your problem. This may include historical records, transactional data, customer interactions, and surveys. You may also want to source some external data to provide additional insights.
The quality and relevance of the data are crucial for making an accurate analysis, which is where the next step comes in.
Data cleaning and preparation
Data should then be cleaned and prepared to ensure accurate and consistent analysis. Raw data often includes errors such as duplicates, missing info, and incorrect formatting.
By removing or correcting these errors your data will be more accurate. For more information on data cleansing read our guide.
Analyze data
Once your data is tidied up it’s time to start analyzing.
There are many different analytic techniques such as exploratory data analysis, statistical analysis, data mining or drilling, and correlation analysis. But you can also make use of AI, machine learning, and other tools to help with your analysis.
Utilizing data analytics tools can help save you time and prevent human error. Just make sure your dataset is secure and your calculations are correct.
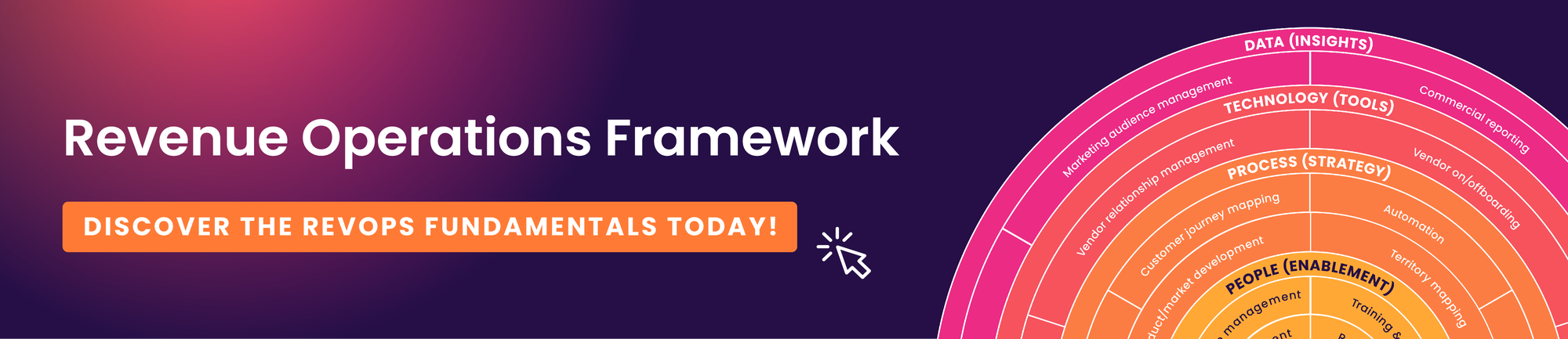
Validate findings
Before taking action on your findings, it’s important to validate the data. Check for statistical significance, scan for any errors, and rerun calculations to ensure the analysis is reliable and any conclusions will be in line with the data.
Reporting and visualization
You may wish to present your findings to key stakeholders, this is where reporting and visualization come in. It’s important to tell a story with the data and ensure it’s easy to follow for your listeners.
Data visualization allows you to easily show complex information in a simple graph or table.
Take action
Once you’ve understood the data and the root cause of your problem, it’s time to brainstorm solutions and take action.
Whether that means reimagining your sales enablement programs, rethinking your SEO strategy, or rewriting your MQL definitions, ensure you take action. After all, diagnostic analytics are only as useful as the actions that come after the data analysis.
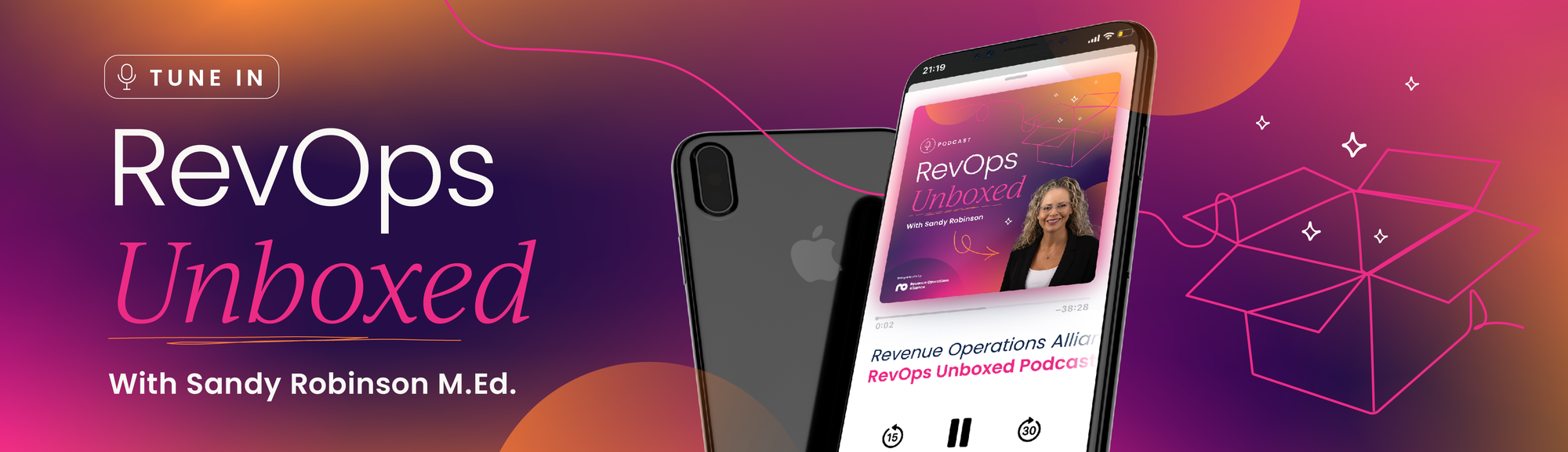
Challenges of diagnostic analytics
Using diagnostic analytics isn’t a miracle solution, there are downsides and challenges associated with its use. Here are three things to consider when using this technique.
Data privacy and security
It’s vital that your organization follows data privacy legal requirements and takes steps to protect your data. Ensure sensitive data is encrypted and any tools you utilize are secure.
It’s also important to follow data management and governance best practices when handling customer data.
Teck stack and human resources
Diagnostic analytics involves a lot of complex data analysis, requiring the appropriate tools to be as efficient as possible. You may need to purchase a data analytics tool if you don’t already have one in your tech stack.
Due to the complexity of the analysis, your team will also have to be trained in the tool and have the skills to carry out the analysis and interpret the data correctly. Or you may want to consider hiring a dedicated analyst.
Biases and subjectivity
While the raw numbers don’t lie, human subjectivity and bias can creep into your analysis at any stage. So it’s important to stay as subjective as possible during this process and gain feedback from others to prevent biased conclusions.
Diagnostic analysis is particularly at risk for confirmation bias, as you may unconsciously use and interpret data in a way that confirms your initial hypothesis.
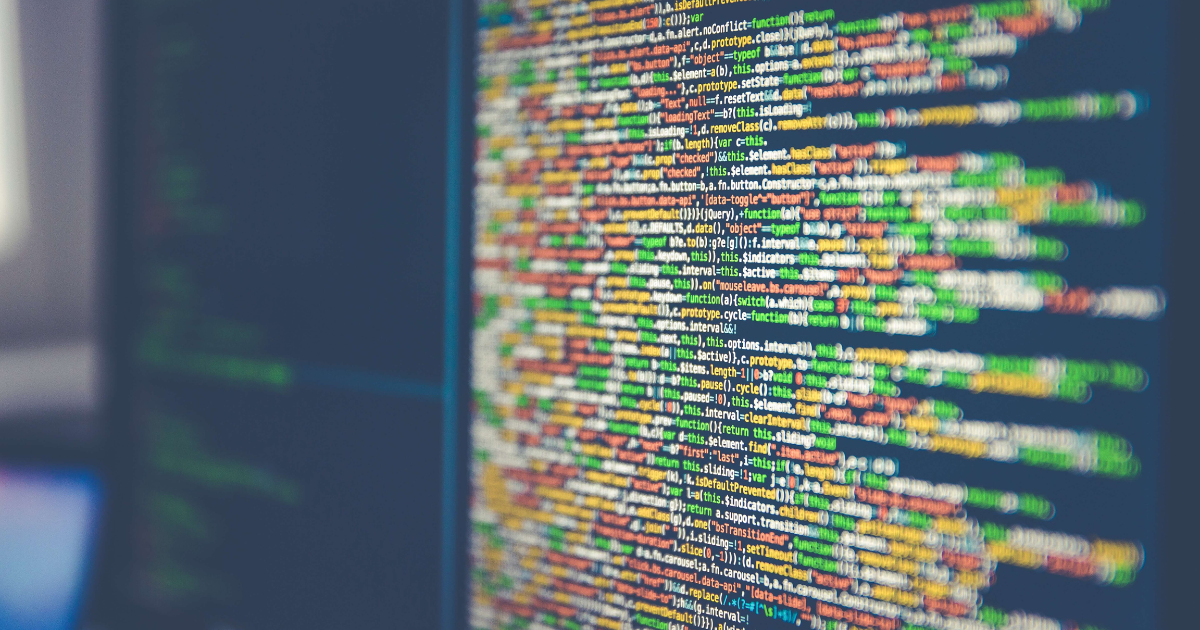
Example use cases of diagnostic analytics for RevOps pros
There are many ways to use diagnostic analytics within your RevOps strategy but here are two common use cases.
Use case 1: Sales spike
Hooray – you’ve noticed a spike in your sales this month! 🥳
But instead of simply hoping your sales numbers will continue to improve month on month, you decide to look further into this month’s success.
Using diagnostic analytics, you can better understand what might’ve caused the spike in sales. In turn, these insights can help you to recreate this success in the future, and set up realistic expectations for next month’s targets.
After diving into the data, you may discover the additional closed-won deals this month were leads from a conference several months ago. This may prompt you to book a stall at the same conference next year.
Or you may discover that the boost in sales has correlated with a new sales enablement program which is showing early signs of success – leading you to invest more money into your enablement team.
Use case 2: Failed marketing campaign
So, your marketing team’s campaign hasn’t brought in as many leads as expected… 😬
There are so many factors to consider when evaluating a marketing campaign’s shortcomings – where should you look first?
Diagnostic analysis can help you answer this question. By taking stock of the campaign data and your historical data, you’ll likely find patterns that point you toward the problem (and allow you to avoid that next time around).
Maybe you’ll discover a seasonal pattern, where leads are less likely to be interested in the summer months (as they tend to take long breaks in the sun). Or perhaps you need to improve your processes for handing leads across to the sales team.
Whatever the reason, using diagnostic analysis can help you to take action and resolve the concern.
Looking for more?
If you enjoyed this article, you’ll love these videos too:
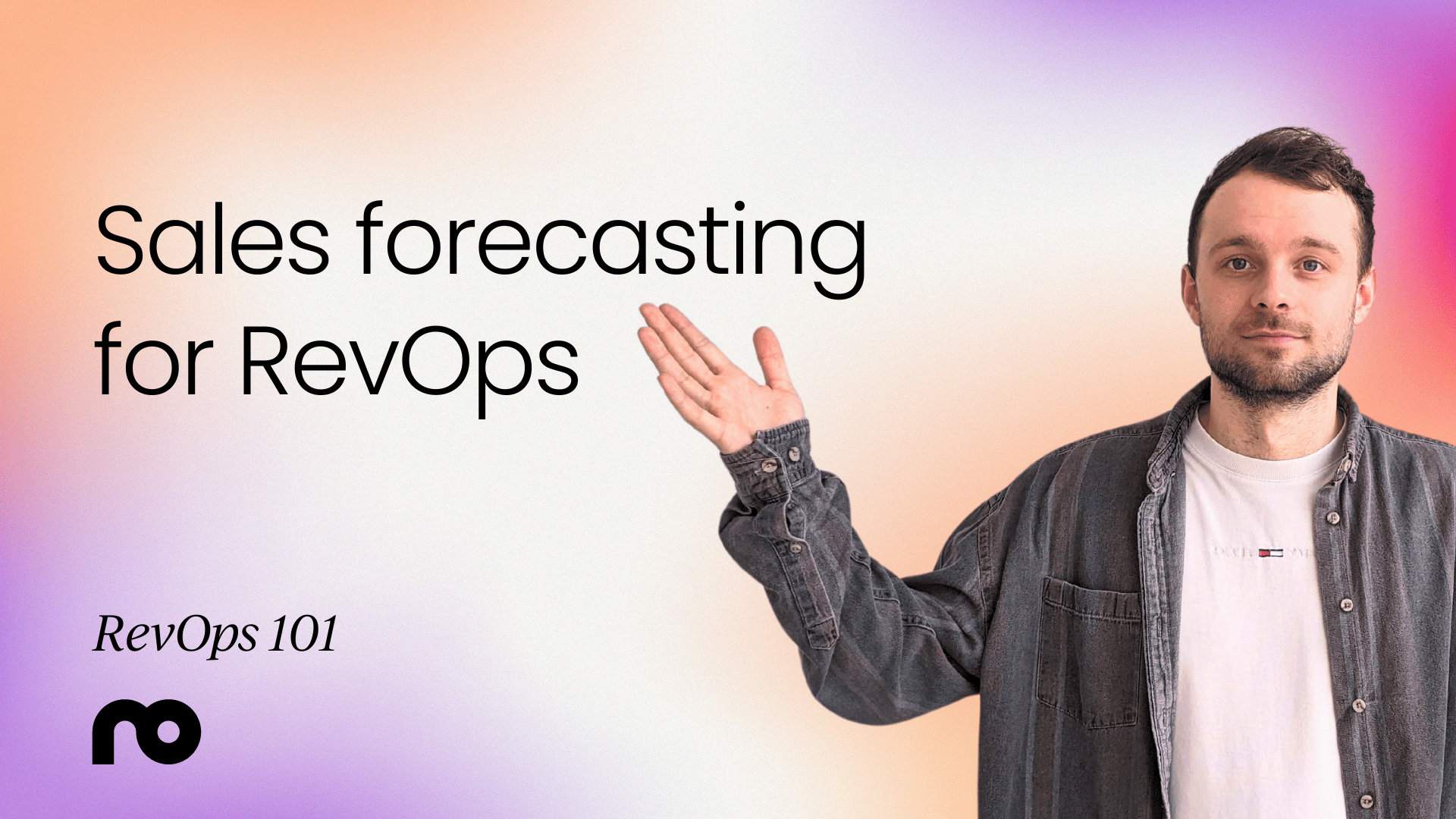
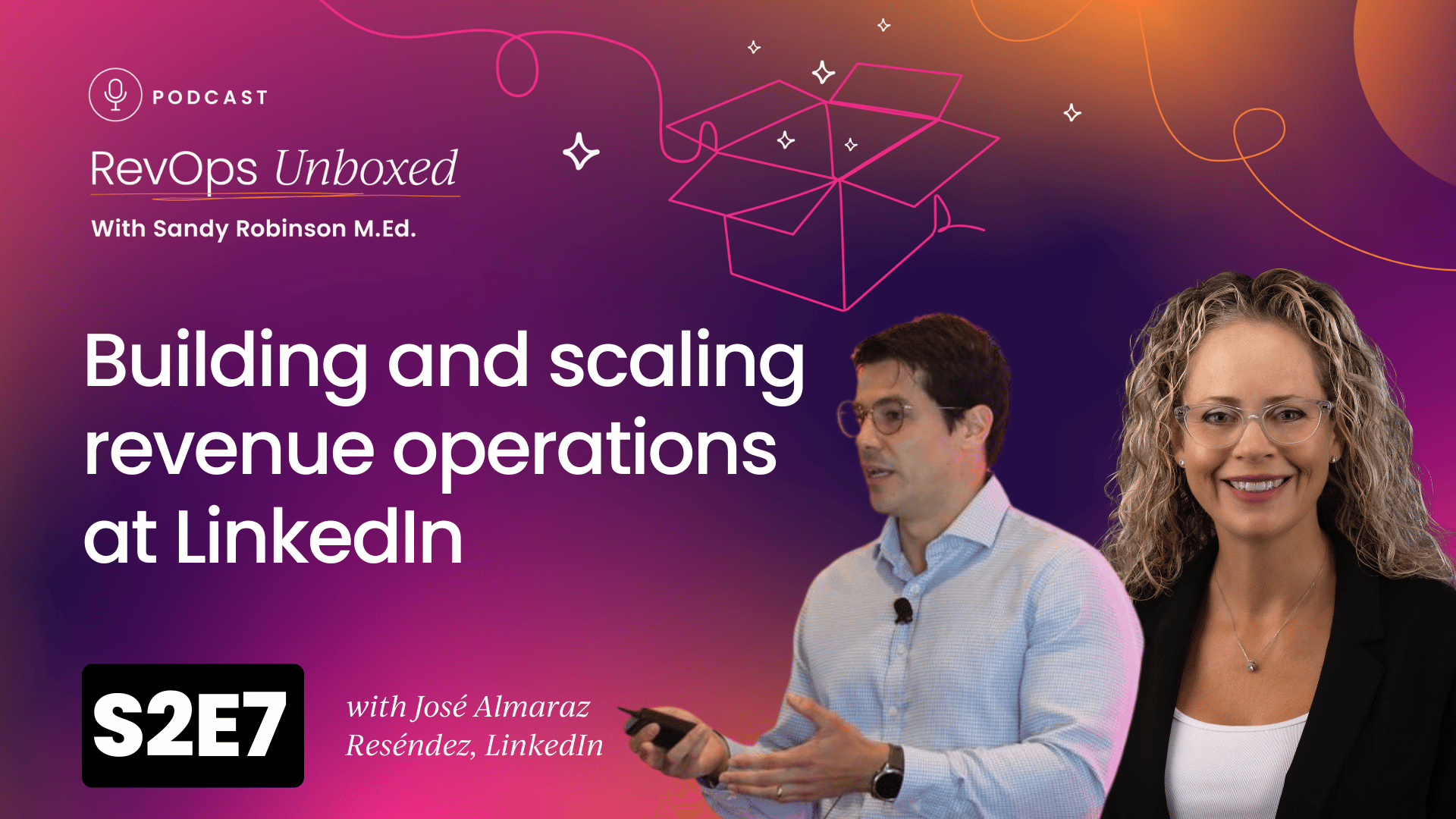