For RevOps professionals, the value of an accurate forecast is self-evident. An accurate forecast:
- Enables proper resource planning for activities downstream from sales
- Allows for strategic pivots to address gaps to budget
- Gives executive leadership and investors confidence that sales has a solid grasp of their business
But how many of us can proudly claim that our teams hit the bullseye more often than the barn side (as one of my fellow ops leaders used to say)?
If you are feeling sheepish, you don’t need to worry. According to Xactly’s 2024 Sales Forecasting Benchmark Report, 42% of sales and finance leaders miss their forecast by more than 10%, with only 20% of leaders obtaining the gold standard of +/- 5% forecast accuracy.
Another report from Miller Heiman Group asserts that 80% of sales organizations miss their forecasts by 25% or more.
So, if you're struggling in this area, you're in good company!
Investing time in improving your forecast accuracy can truly set you apart from the pack as a RevOps professional.
In this article, I show you a step-by-step method for building a robust forecast process that you can improve over time to reach that gold standard of forecast accuracy.
Using this method, I have been able to reach 97% forecast accuracy on day one of the quarter, which, as they say, ain’t too shabby.
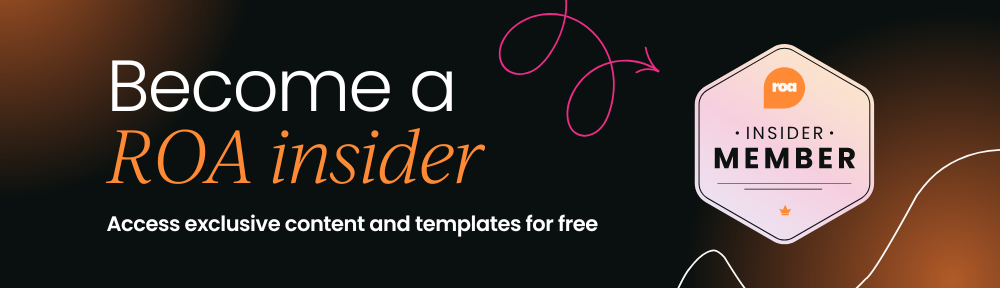
But isn’t there software for that?
Why should you invest the time to build a forecast process when software exists in the market that has solved this problem? There are a few good reasons:
1. Software costs money
You don’t always have money in the budget for software, but you will always be on the hook for an accurate forecast. Building a highly accurate forecast process following this method costs you some time and effort, but no money.
2. Most software can only produce a fairly high-level forecast
If you need a granular forecast by, for example, sales team, market segment, state, and product, most forecasting modules cannot reach that level of granularity.
Those that do are very expensive (see point #1) and take significant effort to implement. The method I outline can be applied to any level of granularity you need in your forecast.
3. Rolling out your own forecast process is the best preparation for implementing forecasting software
Rolling your own forecast process is the best preparation for effectively evaluating, selecting, and implementing the right forecasting software for your business.
By doing it yourself, you'll have a deep understanding of the precise requirements you have for your software vendor.
It's a process, not just a model
Getting to the gold standard of forecast accuracy doesn’t just depend on a sophisticated forecast model.
If you have processes that produce a high degree of variability in your data, it will always be a challenge to produce a highly accurate model.
We're all tempted to smooth out variability in the data with analytical techniques, but no amount of Excel or BI gymnastics will produce the same impact as processes that produce a reliable standard of quality.
Define your sales process
Since we are going to be doing math on Opportunity Created Dates, Close Dates, Stages, and Forecast Categories, we need to make sure we have aligned with leadership, documented, and enabled our sales teams on:
- When to open an opportunity?
What are the qualification criteria for opening an opportunity?
- When to close an opportunity as lost?
How many days without activity do we tolerate before the close the opportunity as lost?
What are the entry and exit criteria for opportunity stages and forecast categories?
What are the client actions that justify setting a given stage or forecast category?
Answering these questions at a minimum removes a ton of subjectivity from the sales process, and dramatically improves the consistency of the elements that we will analyze in the forecast (pipeline conversion and forecast pipeline generation).
The last point about using client actions is particularly important, since selecting the right stage and setting the right forecast category is, in the absence of clear criteria, one of the most subjective aspects of the sales process and therefore the most vulnerable to high variability.
We want facts over feelings.
Here is an example of how I worked with our leaders to define specific client actions that should determine the forecast category of the opportunity:
Determine your grain
Identifying the correct inputs to your forecast model and ensuring the data quality of those inputs in your forecast process requires understanding the level of granularity that you need for your forecast.
That is, which dimensions do you need to measure (sales team, product, forecast range, one-time revenue vs. recurring revenue, etc.)?
Do not fall into the temptation of forecasting at the most granular level possible to anticipate all possible applications of the forecast.
There are two reasons for this:
- The more granular your forecast is, the more up-front lift is required to set up your process and model. This will delay time to value for your effort.
- Forecast accuracy decreases as the level of granularity increases. You will always be more accurate at higher levels of abstraction.
The point is, as always, to measure what matters.
What is the level of granularity you need that will create impactful insights that drive business decisions? Nice-to-know is not a sufficient criterion.
Align with the key stakeholders of the forecast (sales & marketing leadership, implementation, finance, etc.) on the level of granularity you need before you start and document the decision.
If you are just getting a forecast process started at your organization, I recommend starting at a high level of abstraction – such as forecasting by sales team, quarter, and total bookings amount – and then refining in future iterations only if you determine, in alignment with your stakeholders, that additional detail would provide additional value.
Don’t try to build the crystal palace from the get-go, but build a solid house, brick by brick.
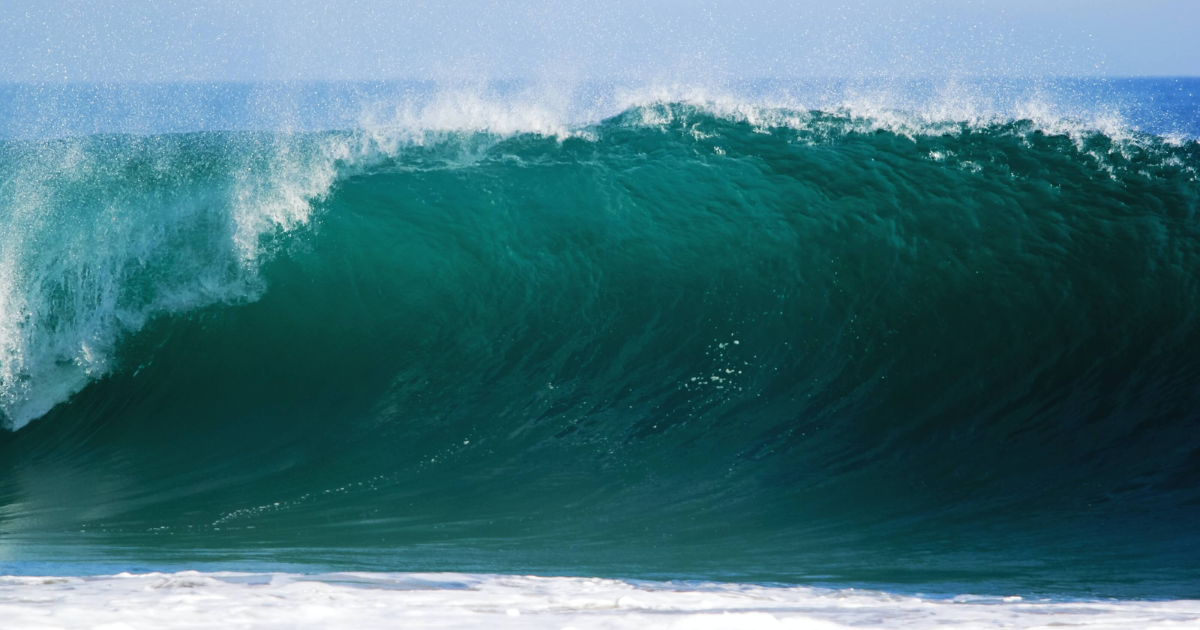
It’s elementary, Watson
We are going to get into the brass tacks of building the process in a moment, but before doing so, it is helpful to step back and understand what the core elements of this forecast process are.
There are four elements across two categories that you will use to build your process:
- Open Pipeline, consisting of
- Committed Pipeline
- Best Case Pipeline
- Pipeline Pipeline (not a typo, I promise)
and
- In-Period Created and Closed (IPCC) Pipeline
At the highest level, the process looks at the conversion of your existing pipeline in each forecast category (I use the standard, Commit, Best Case, and Pipeline categories above) and how much pipeline will be created and closed won in the forecast period.
The reason it's helpful to understand the elements of your forecast process is because, however granular you may need to make your forecast, you are repeating the same steps to combine these same elements.
If you're forecasting total bookings at the team level by quarter, you need to derive these elements and combine them at that level.
If you're forecasting ARR vs. Implementation at the team level by quarter, you need to derive the same elements, but with the added dimension of recurring vs. non-recurring revenue.
The elements stay the same!
Model 1: Forecast total bookings by team and quarter
Model 2: Forecast ARR vs. Implementation by team and quarter
Snapshot your pipeline
So, let’s get into it. To show you the principles behind building a highly accurate forecast process, I'll walk you through building a simple model to forecast total bookings by team for the quarter.
It all starts with getting as much relevant historical data on transformations in your pipeline as possible.
The “relevant” adjective is important, since the accuracy of your forecast depends on a broad-based similarity of your go-to-market teams over time.
If, for example, you fundamentally reorganized the teams at the beginning of last year, data from before the reorganization will most likely not contribute to improving the accuracy of your forecast.
If you do not have a process to snapshot the pipeline in place already, do so as soon as possible.
You can work with your business intelligence department to do this in a data warehouse, you can pull pipeline data into a BI tool yourself and use R or Python to write it out on a periodic basis, or you can run quick and dirty and have a calendar reminder to export the pipeline.
Since most teams forecast on a weekly basis, aim to snapshot at least weekly and always on the same day to create consistency.
Include in your snapshot all opportunities from the current period and onward and ensure all the fields in your snapshot align with the desired granularity of your forecast.
Ideally you'll have at least 12 months of snapshots to work with to account for seasonality in how your pipeline changes over time, but you don’t need that much to get started.
Just know that your model’s accuracy will grow in step with the amount of historical data you have available.
You can start with even one month of snapshots, since understanding how the pipeline changes week by week even in one month will represent a gain in accuracy vs. nothing if you were not snapshotting before.
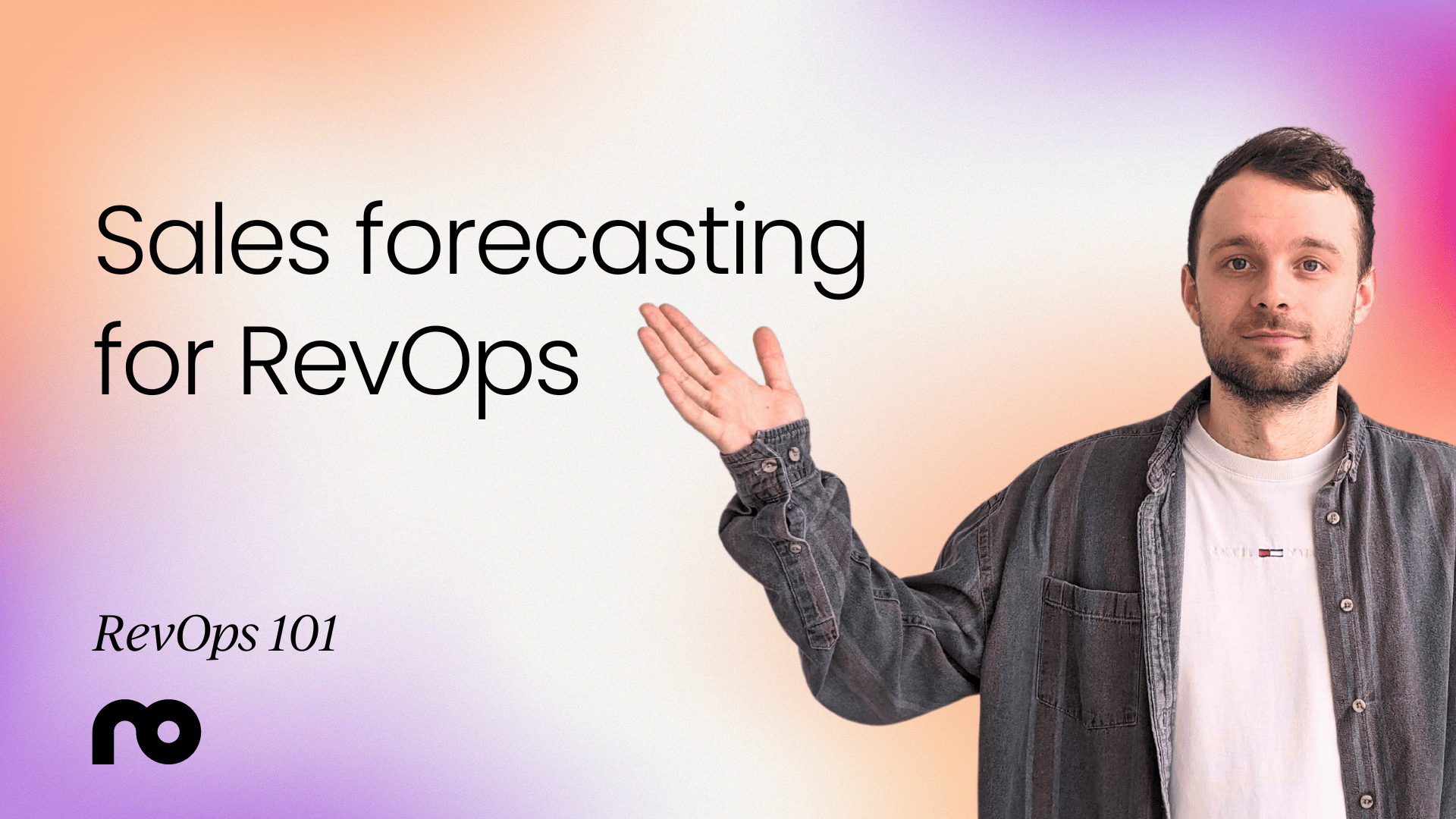
Gather your forecast elements
Using your weekly snapshot data, it is time to calculate your forecast elements: pipeline conversion by forecast category and in-period created and closed won.
You can work on all of your snapshots as different tabs in a spreadsheet or, if you have learned a bit of BI, can combine them into a single table using Power Query, for example.
In your snapshot data, in addition to your CRM fields, you need to add fields (either calculated columns in a BI solution or table columns in Excel) for:
- Snapshot Date: the date on which you took the snapshot
- Snapshot Forecast Week: the week in the month for which you took the snapshot. We will be calculating conversion metrics by week so that we can reforecast each week.
Now you need to enrich your snapshot data with current opportunity data, since we need to know what happened to those opportunities after the snapshot was taken.
Once again, you can simply export a current view of pipeline including the same fields as in your snapshot or, with a bit more effort, query the data directly from your CRM into your BI tool of choice.
Using your current opportunity data, add look-up columns to your snapshot data for:
- Current Stage
- Current Close Date
- Current Forecast Category
- Current Amount
So far, your snapshot data will look like this:
Next, add a few helper columns that will enable us to calculate the conversion metrics:
- Snapshot Date (YYYY-QQ): the year and quarter of the Snapshot Date. We'll use this in conjunction with the Snapshot Close Date (YYYY-QQ) and the Current Close Date (YYYY-QQ) to isolate pipeline that, as of the snapshot date, was expected to close in the quarter and that actually did close in the quarter. Getting the quarter number is relatively easy in BI tools, but if you need a bit of help to do this in Excel, take a look at these resources from Exceljet: Get quarter from date or Get fiscal quarter from date.
- Snapshot Close Date (YYYY-QQ): the year and quarter of the Snapshot Close Date.
- Current Close Date (YYYY-QQ): the year and quarter of the Current Close Date.
- Pipeline in Period: checks if Snapshot Date (YYYY-QQ) is equal to Snapshot Close Date (YYYY-QQ). We will use this as a filter to isolate the pipeline from the snapshot that had an impact to the current forecast period.
- Converted: checks if the Snapshot Close Date (YYYY-QQ) is equal to the Current Close Date (YYYY-QQ) and the Current Stage = Closed Won. This identifies the pipeline that was expected to close in the current period that in fact did close in the current forecast period. By combining this with Pipeline in Period, we can find the pipeline that had an impact to the current forecast period that was expected to close that in fact did close.
Your complete table will now look like this:
I input some sample data to illustrate how the different columns help you to find the conversion.
The first row, where Pipeline in Period is TRUE and Converted is TRUE represents pipeline that converted from Best Case (Snapshot Forecast Category) to won in the forecast period. Row two did not convert. Row three did not convert and the pipeline was not in the forecast period. Row four converted, but was not part of the forecast period.
I find that it is clearest to use two pivots: one with the snapshot pipeline value (Snapshot Amount), which is your denominator for the conversion metric; the other with the current pipeline value (Current Amount), which is the numerator for the conversion metric.
The example below shows the pivots set up to calculate conversion metrics for week one in the quarter using all available snapshot data for week one.
With the two pivot tables set up, you get your conversion metric for each forecast category by dividing the Converted = TRUE values from the pivot with the Current Amount (highlighted in purple) by the Total from the pivot with the Snapshot Amount (highlighted in orange).
In this way, you not only know how much of the original snapshot value pipeline converted, but you also account for changes in pipeline value on the journey to closed. Nifty!
If you want to look at conversion metrics for a specific snapshot, you can add the Snapshot Date to the filters.
If you want to see how conversion metrics have been changing over time for week one of the forecast period to assess the degree of variance, you can add the Snapshot Date to the rows in the pivot.
In short, because of the way we have structured the inputs to the forecast metrics, you have a ton of flexibility in examining those metrics.
This flexibility will be important when we pilot the model and make adjustments to it to improve its accuracy.
With the conversion metrics complete, we just need to calculate the in-period created and closed (IPCC) pipeline.
You can use your snapshot data to calculate this as well, but I find it is cleaner simply to do a fresh pull (report or query) of pipeline generation data across your historical range.
Once you have that extract, since, in this example, we are looking for pipeline that was created and closed won in the same quarter, add the following columns to your dataset:
- Created Date (YYYY-QQ): the year and quarter of the opportunity Created Date.
- Close Date (YYYY-QQ): the year and quarter of the opportunity Close Date.
- IPCC Converted: checks if Created Date (YYYY-QQ) is equal to Close Date (YYYY-QQ) and if Stage = Closed Won.
Your complete table will look like this:
In the first row IPCC Converted = TRUE because the Created Date and the Close Date are in the same quarter and the Stage = Closed Won.
In row two, IPCC Converted = FALSE since the Created Date and Close Date are in different quarters, even though the opportunity is Closed Won.
In row three, IPCC Converted = FALSE since the Stage is not Closed Won, despite the fact that the Created Date and the Close Date are in the same quarter.
Using this data, we can create the following pivot to summarize in-period created and closed pipeline by team and quarter:
Each time you reforecast, you will adjust the Created Date filter to remove days that have already transpired in the forecast period you are analyzing, so as not to inflate the in-period created and closed amount.
For example, if you are reforecasting Q2 on April 7, you will remove April 1-6 from the Created Date filter; otherwise, you will be inflating the IPCC number in your analysis.
Forecast … assemble!
With our elements complete, we can now create the forecast analysis.
Most of the heavy lifting is behind us at this point and we are now just putting the elements together. As a reminder, in our example we are assembling a total bookings forecast by team for the quarter.
The first step in assembling the forecast is to extract the current open pipeline for the quarter.
We will now combine the current open pipeline data, the conversion metrics for each forecast category, and the in-period created and closed pipeline in a summary table to produce the forecast.
This will tell us how the pipeline that already exists in the forecast period will convert to bookings as well as how any pipeline that is creating during the forecast period will convert to bookings.
Your summary table will look like this:
- $ Won Bookings: the sum of Amount on Closed Won Opportunities.
- $ Commit Pipeline: the sum of Amount on Opportunities with Forecast Category = Commit.
- % Conversion (Commit): the conversion element we calculated above for Forecast Category = Commit.
- $ Conversion (Commit): returns the % Conversion (Commit) times the $ Commit (Pipeline) to yield the Amount of pipeline currently in Forecast Category = Commit that is anticipated to convert to bookings.
- The same definitions apply to the Best Case and Pipeline forecast categories in the adjacent columns, for those respective forecast categories.
- IPCC: the in-period created and closed element we calculated above.
- $ Forecast: the sum of the $ Won Bookings, $ Conversion (Commit), $ Conversion (Best Case), $ Conversion (Pipeline), and IPCC (highlighted in purple). This is the forecast we have been working so hard to calculate!
That’s it! You put in the hard work to calculate the forecast elements. The last step was just to bring them together in a harmonious symphony of data-driven accuracy.
Repeat this process weekly, deriving the elements using the corresponding week number from the Snapshot Data.
For example, in the second week of the quarter, you would use the conversion metrics from the pivot for Forecast Week = 2 as well as remove any days from the IPCC metric that had already transpired by the time of the reforecast.
Control, monitor, improve
To maintain and improve the accuracy of your forecast, you need to understand and control the elements that make it up.
That means documenting your specific process to ensure the elements are produced the same way each time.
This is especially important if you have a team working on producing the forecast or if you are handing the forecast process off to another team member.
In this way, if your accuracy is suffering, you know that it is not due to variation in your process, but rather a deficiency in one of the elements that you may need to re-examine.
How do you know if you need to re-examine one or more elements?
You need to compare forecast with actuals after every forecast period is completed for each of the elements (Commit Conversion, Best Case Conversion, Pipeline Conversion, and IPCC) and for each weekly reforecast.
If any of these elements begin to fall outside the gold standard of +/-5% forecast accuracy, you may need to examine your historical periods to determine if you are including periods that represent significant outliers compared to the overall trend.
Outliers will skew the elements and throw off your forecast accuracy.
When the historical dataset you use is not extensive, outliers will have an outsize impact on accuracy; however, as the size of the historical dataset grows, the elements tend to become more stable.
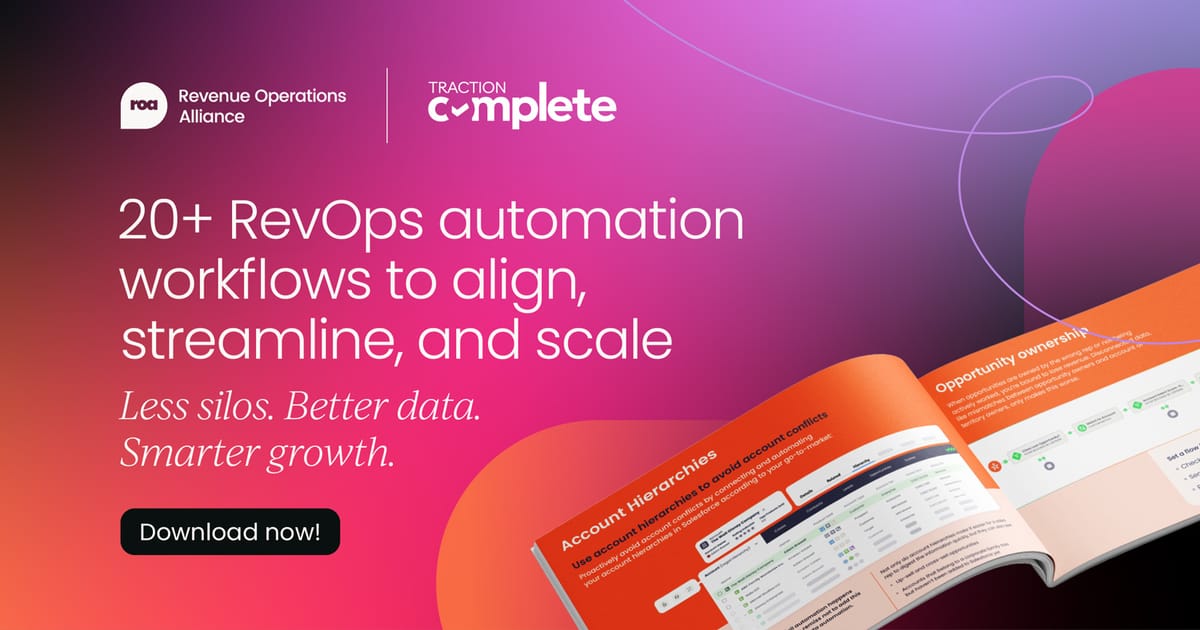
But is past always prologue?
Since this model relies heavily on historical data to predict future performance, you might ask what happens when the present stops looking like the past?
What happens when we expand headcount? Won’t that increase IPCC? What about adopting a new sales methodology? Shouldn’t that improve conversion rates?
The answer, of course, is yes.
But that doesn’t mean it is time to abandon this model. That means it is time to go deeper on our elements.
If we have new headcount, we can use the historical data, combined with distinct counts of opportunity owners, to figure out the IPCC rate per headcount.
We can then increase the IPCC rate in the model by the IPCC rate per headcount times the number of new headcount (and even add a ramp percentage if the reps are not yet fully ramped).
Similarly, we can chart conversion rates over time to monitor their variation and make educated inferences about their future trajectory if a new sales methodology is installed.
We can also make conservative assumptions of the impact on conversion rates using industry benchmarks and assess the accuracy of the model using those assumptions.
Elemental power
The power of this model is the power of abstraction that the elements provide.
Understanding the extent to which the past is representative of the present and how present factors may create differences to past conditions is essential to creating an accurate forecast, but the elements remain your steady guide as you travel from past to present and future.
Embrace the elements, and you will be well on your way to a consistently accurate forecast.
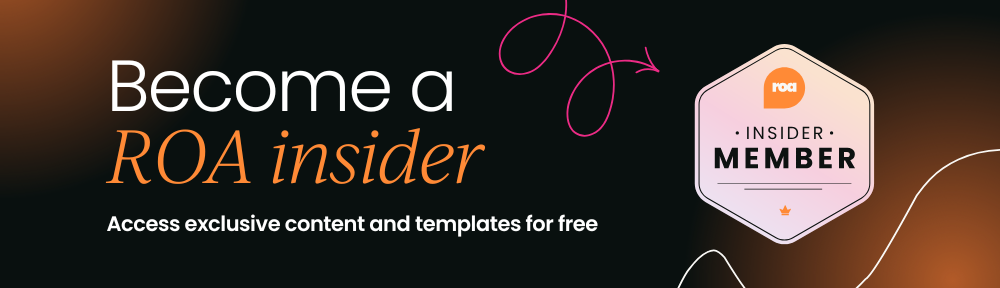